Lecturer: Andreas Kalckert
Fields: Cognitive neuroscience; Experimental psychology
Content
In this course I will provide an overview of the cognitive, perceptual, and neural mechanisms underpinning bodily self-awareness. Following Gallagher’s distinction of ownership vs. agency and using the rubber hand illusion as an experimental model, I will discuss how sensory and motor processes give rise the experience of the own body.
The course consists of two talks introducing the experimental and theoretical findings, followed by a hands-on session with the rubber hand illusion demonstrating some of the practical caveats when performing this experiment.
Literature
- Ehrsson HH. Multisensory processes in body ownership. In: Sathian K, Ramachandran VS, eds. Multisensory Perception: From Laboratory to Clinic. Academic Press: Elsevier; 2020:179-200.
- Graziano, M. S. A., & Botvinick, M. (2002). How the brain represents the body: insights from neurophysiology and psychology (pp. 136–157). In: Common Mechanisms in Perception and Action: Attention and Performance XIX. Eds. W. Prinz and B. Hommel. Oxford University Press, Oxford England
- Kalckert, A. (2017) I am moving my hand – Ownership, Agency, and the body. in “Sensation of movement”. Ed. Thor Gruenbaum and Mark Schram Christensen. Psychology Press: Current Issues in Consciousness Research
- Kalckert, A. & Ehrsson, H.H. (2012). Moving a rubber hand that feels like your own: a dissociation of ownership and agency. Frontiers in Human Neuroscience, Volume 6, Article 40
Lecturer
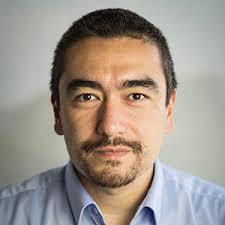
Dr. Andreas Kalckert received his Ph.D. from the Karolinska Institute, Sweden (Brain, Body, and Self Lab, Department of Neuroscience). After his Ph.D. he worked as a lecturer in psychology at the University of Reading Malaysia. He works now as a Senior lecturer in Cognitive Neuroscience at the Department of Cognitive Neuroscience and Philosophy at the University of Skövde (Sweden). In his research, he specializes in the cognitive and perceptual processes of bodily self-awareness. His particular interests lies in the interaction of the Sense of Ownership and Sense of agency in body illusion paradigms such as the rubber hand illusion.
Affiliation: Department of Cognitive Neuroscience and Philosophy, University of Skövde (Sweden)
Homepage: https://www.his.se/en/research/systems-biology/cognitive-neuroscience-and-philosophy/