Lecturer: Erika Covi
Fields: Emerging memory devices, Neuromorphic computing
Content
In recent years, the cloud-based approach to data classification has been challenged by the edge computing paradigm, which has enabled real-time data processing at the network edge, ideally next to the sensor collecting data. This paradigm poses severe constraints on the systems in terms of power-efficiency, compactness, and latency [1, 2]. Therefore, we need to explore unconventional hardware solutions able to meet these stringent requirements.
Brain-inspired architectures, particularly Spiking Neural Networks (SNNs), are promising candidates to achieve low-latency computation, and stateful, energy-efficient operations [3]. However, their current implementations primarily rely on digital or mixed-signal Complementary Metal-Oxide-Semiconductor (CMOS) technologies, which pose challenges in meeting the demanding memory, area, and power constraints of computing on the edge [1].
In this context, the integration of embedded memristive technologies holds a significant promise to enhance the capabilities of CMOS technology [2, 4] for the development of neuromorphic hardware [5]. Memristive devices are nanoscale devices able to change their conductivity upon application of proper electrical stimuli. They offer fast and energy-efficient tuneable volatile and non-volatile storage, and are therefore well-suited for storing SNN parameters. The exploitation of their unique properties, such as operation voltages compatible with current CMOS technology as well as analogue, neural-/synaptic-like behaviour, offer an attractive opportunity for realizing energy-efficient and massively parallel computing architectures in conjunction with CMOS technology [3, 5]. Indeed, these features enable efficient computation, neural dynamics, and synaptic plasticity, which are essential traits for emulating the brain’s functionality in hardware [4, 6].
In this course, we explore the role of memristive devices in emulating the functionality of neural networks, enabling edge systems to classify and recognise patterns or process sensory inputs. We emphasize the need for co-developing memristive devices with CMOS circuits to enable seamless integration and to exploit the strengths of both technologies. Furthermore, we discuss how the co-development of memristor devices, CMOS circuits, and innovative learning algorithms can facilitate edge computing paradigms. Moreover, the intrinsic physical characteristics of memristive devices, if correctly exploited, enable analogue computing, thus offering a compelling alternative to traditional digital approaches. We also discuss the challenges and opportunities of developing memristive-CMOS hardware neuromorphic architectures. The co-design of devices, circuits, and algorithms indeed requires to identify and address issues related to device variability, scalability, and system integration.
In conclusion, the synergistic co-development of memristive devices, CMOS circuits, and innovative algorithms can pave the way for intelligent edge devices capable of performing complex cognitive tasks.
Literature
- [1] E. Covi et al. Front. in Neurosci., 15, 611300 (2021).
- [2] D. V. Christensen et al. Neuromorph. Comput. Eng., 2, 022501 (2022).
- [3] E. Chicca et al. Proc. of the IEEE, 102, pp. 1367-1388 (2014).
- [4] D. Ielmini and S. Ambrogio, Nanotech., 31, 092001 (2019).
- [5] A. Amirsoleimani et al. Adv. Intell. Sys., 2, 2000115 (2020).
- [6] E. Covi et al. Neuromorph. Comput. Eng., 2, 012002 (2022).
Lecturer
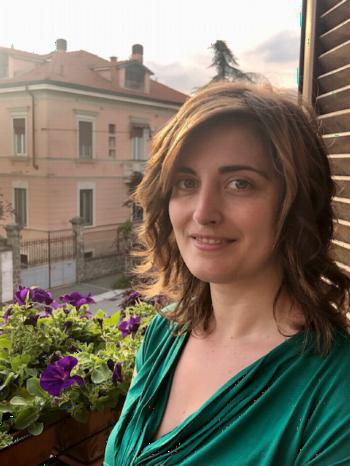
Dr. Erika Covi is Assistant Professor at the Zernike Institute for Advanced Materials & Groningen Cognitive Systems and Materials Center (Groningen, the Netherlands). She received her PhD in Microelectronics in 2014 from the University of Pavia (Italy), where she worked on designing integrated systems for the characterisation of memristive devices. She also worked at the National Research Council (CNR) of Italy, at Politecnico di Milano (Italy), and at NaMLab gGmbH (Dresden, Germany). Her research interests lie at the intersection of emerging devices, circuit design, and brain-inspired computing. More specifically, they focus on exploiting the intrinsic physical characteristics of memristive devices to reproduce computational primitives of the brain in mixed neuromorphic-memristive systems.
Affiliation: University of Groningen