Lecturer: Alexander Hölken
Fields: Dynamical Systems Theory, Complexity Theory, Causality, Cognitive Science
Content
One of the central questions of cognitive science and neuroscience is that of how an organism’s intracranial states cause, and are in turn affected by, their behavior. Within cognitive science in particular, there exists a long-standing tradition of analyzing processes of cognition and behavior in terms of linear chains of cause and effect. For instance, computational theories of mind generally assume that the brain (or parts of it) operate similar to, or literally are, discrete-state machines (Chalmers, 2011). Thus, computational explanations of how cognitive processes cause behavior often refer to brain states as discrete entities, whose instantiation at some timestep t causes the instantiation of another discrete state at t+1, and so on, eventually realizing the behavior in question. While these assumptions are well-suited for explaining the development of abstract computational systems, they often fail when applied to real-life biological systems. This is because these systems don’t operate analogously to discrete-state machines:
1. Discrete-state systems are neatly decomposable into spatially separate entities that cause each other to change from one discrete timestep to the next. This need not be the case for complex natural systems (Kelso & Engstrom, 2008; Eigen, 2013; Lamb, 2015).
2. In discrete-state systems, temporality matters only in regards to the order in which system states happen in. In complex natural systems, both order and timing are causally relevant.
3. In discrete-state systems, the part/whole relationship is a mechanistically reductive one: The development of their larger-scale parts is explicable purely by reference to states of smaller-scale parts, along with their position on a causal chain. In complex natural systems, processes on the level of the whole system can causally influence processes on lower levels. This makes mechanistic reduction impossible: Even complete descriptions of lower-level interactions are not sufficient to explain the development of the whole (Bishop, 2008).
In this course, we will learn about the unique causal features of complex natural systems and how they explain their development. In particular, we will look at the relationship between wholes and their parts, and how system states at different levels constrain each other over time. In order to do so, we will become familiar with the concepts of dynamical regimes, attractor landscapes, and order/control parameters (Kelso, 1995) by looking at simple examples which do not presuppose a mathematical background. Many of these examples will come from experiments that explicitly aim to manipulate e.g. the control parameters of a dynamical regime, for instance in psychological studies about behavioral coordination (Schöner, Zanone & Kelso, 1992; Buchanan, 2004; Nalepka et al., 2021). Finally, we will wrap up by discussing possible solutions for contemporary problems that this methodology may offer, such as the creation of artificial general intelligence.
Literature
- Bishop, R. C. (2008). Downward causation in fluid convection. Synthese 160, 229 – 248. DOI: 10.1007/s11229-006-9112-2
- Buchanan, J. J. (2004). Learning a single limb multijoint coordination pattern: the impact of a mechanical constraint on the coordination dynamics of learning and transfer. Experimental Brain Research 156, 39 – 54. DOI: 10.1007/s00221-003-1763-3
- Chalmers, D. J. (2011). A Computational Foundation for the Study of Cognition. Journal of Cognitive Science 12, 323 – 357.
- Eigen, M. (2013). From Strange Simplicity to Complex Familiarity. Oxford University Press. • Kelso, J. A. S. (1995). Dynamic Patterns: The self-organization of brain and behavior. The MIT Press.
- Kelso, J. A. S. & Engstrøm, D. A. (2008). The Complementary Nature. The MIT Press.
- Lamb, M. (2015). Characteristics of Non-reductive Explanations in Complex Dynamical Systems Research. [Doctoral Thesis, University of Cincinnati]
- Nalepka, P., Silva, P. L., Kallen, R. W., Shockley, K., Chemero, A., Saltzman, E. & Richardson, M. J. (2021). Task dynamics define the contextual emergence of human corralling behaviors. PloS ONE 16, e0260046. DOI: 10.1371/journal.pone.0260046
- Schöner, G., Zanone, P. G., Kelso, J. A. S. (1992). Learning as Change of Coordination Dynamics: Theory and Experiment. Journal of Motor Behavior 24, 29 – 48
Lecturer
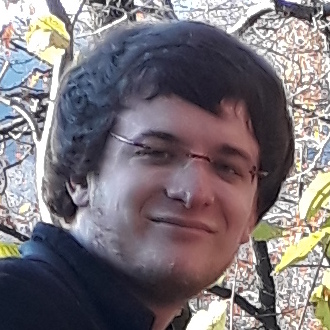
Alexander Hölken received his M.Sc. in Cognitive Science in March 2022 and is currently working on his PhD at the Ruhr-University Bochum. His research focuses on Dynamical Systems approaches to explaining cognitive behavior as a result of the coordination of intracranial states and processes with states and processes of the body and the environment. His doctoral thesis aims to develop a non-reductive physicalist theory of how mental states/processes can be genuinely causally efficacious.
Affiliation: Ruhr-Universität Bochum