Lecturer: Alexandra Kirsch
Fields: Artificial Intelligence
Content
Intuity combines strategy, creativity, design, science, and technology in one place. We deal with numerous interconnected and ever changing pieces of information, for instance snippets from a requirement workshop with users, customers or product owners of a new service, product or software application. This is why we set out to build our own tool for exploring, consolidating and structuring knowledge.
From a user perspective Sort-it helps to solve complex problems. Under the hood Sort-it implements a novel knowledge representation approach that combines classical frame-like knowledge representation with cognitive models of human categorization.
Sort-it is a prime example demonstrating that AI is more than data science and that AI techniques are only as powerful as their application to real-world user demands.
Literature
- https://www.intuity.de/en/blog/2020/wie-man-mehr-aus-workshops-herausholt/
- George Lakoff: Women, Fire and Dangerous Things. University of Chicago Press, 1987
- Herbert A. Simon: The Architure of Complexity. Proceedings of the American Philosophical Society, Vol. 106, No. 6. (Dec. 12, 1962), pp.467-482
Lecturer
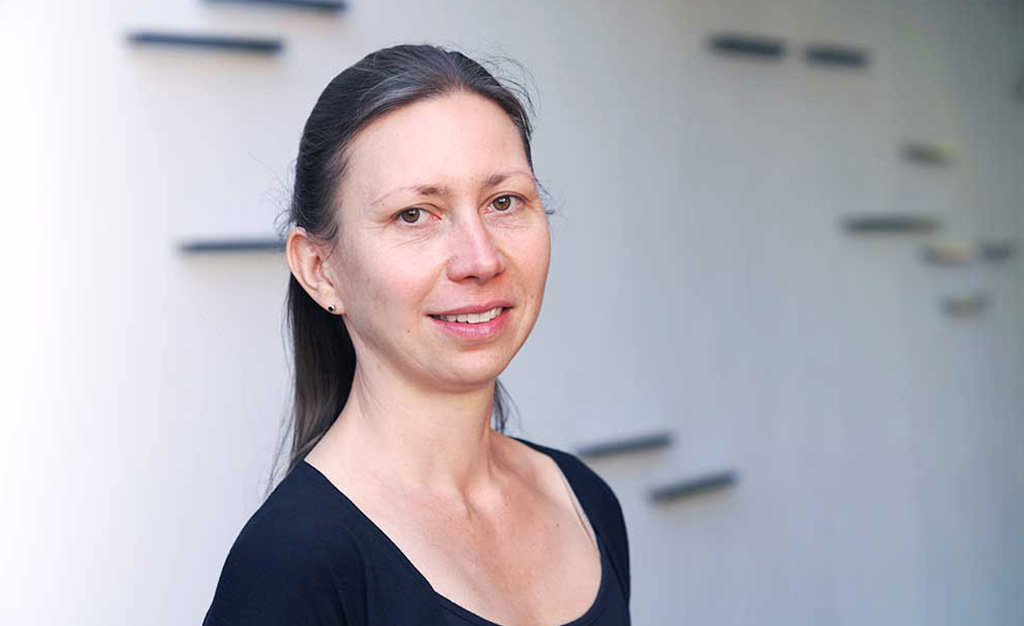
Alexandra Kirsch received her PhD in computer science at TU München. She gathered experience as a business consultant before returning to TU München as a junior research group leader in the cluster of excellence “Cognition for Technical Systems”. She was a Carl-von-Linde Junior Fellow of the Institute for Advanced Study of TU München and member of the Young Scholars Programme of the Bavarian Academy of Sciences and Humanities, where she could profit from a broad interdisciplinary exchange. She was appointed assistant professor at the University of Tübingen for the area of Human-Computer Interaction and Artificial Intelligence. Since 2018 Alexandra Kirsch has been exploring and prototyping applications that combine Artificial Intelligence with User Experience Design at Intuity Media Lab.
Affiliation: Intuity Media Lab GmbH
Homepage: https://www.intuity.de/, https://www.alexkirsch.de/