Lecturer: Ivana Kajic
Fields: Artificial Intelligence, Machine Learning, Cognitive Science
Content
Recent years have seen a proliferation of machine learning models that are capable of producing high-quality images that faithfully depict concepts described using natural language. Such models can generate images that represent arbitrary objects, object attributes, and complex relations between objects. In this talk, I will show that despite these impressive advancements, such models can still struggle with relatively simple tasks. Specifically, I will demonstrate that even the most advanced models have only a rudimentary notion of number sense. Their ability to correctly generate a number of objects in an image is limited to small numbers, and it is highly dependent on the linguistic context the number term appears in. I will further highlight challenges associated with evaluation of different model capabilities, including evaluation of numerical reasoning, and talk about different automated approaches that can be used to evaluate models in a more interpretable way by leveraging existing tools in machine learning and cognitive science.
Literature
- Kajić, I., Wiles, O., Albuquerque, I., Bauer, M., Wang, S., Pont-Tuset, J., & Nematzadeh, A. (2024). Evaluating Numerical Reasoning in Text-to-Image Models. 38th Conference on Neural Information Processing Systems.
- Testolin, A., Hou, K., & Zorzi, M. (2024). Large-scale Generative AI Models Lack Visual Number Sense. arXiv preprint arXiv:2402.03328.
- Wiles, O., Zhang, C., Albuquerque, I., Kajić, I., Wang, S., Bugliarello, E., Onoe, Y., Knutsen, C., Rashtchian, C., Pont-Tuset, J. & Nematzadeh, A. (2024). Revisiting Text-to-Image Evaluation with Gecko: On Metrics, Prompts, and Human Ratings. arXiv preprint arXiv:2404.16820.
- Kajić, I., & Nematzadeh, A. (2023). Evaluating Visual Number Discrimination in Deep Neural Networks. In Proceedings of the Annual Meeting of the Cognitive Science Society (Vol. 45, No. 45).
- Nieder, A. (2020). The adaptive value of numerical competence. Trends in Ecology & Evolution, 35(7), 605-617.
- Dhariwal, P., & Nichol, A. (2021). Diffusion models beat GANs on image synthesis. Advances in neural information processing systems, 34, 8780-8794.
Lecturer
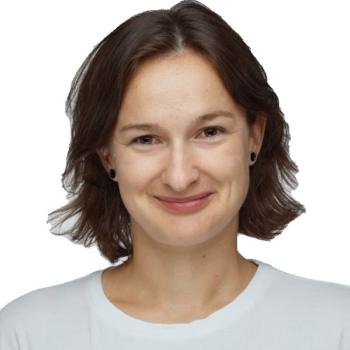
Ivana Kajić is a Senior Research Scientist at Google DeepMind in Montréal, Canada. Her research interests include applying methods and techniques from cognitive science in analysis and characterization of behavior of machine learning models. Specifically, this includes designing evaluation protocols, benchmarks and metrics to comprehensively understand capabilities and limitations of large vision-language models that in recent years have demonstrated strong performance in a variety of tasks. She completed her PhD thesis titled “Computational Mechanisms of Language Understanding and Use in the Brain and Behaviour” in 2020 at the University of Waterloo in Canada.
Affiliation: Google DeepMind
Homepage: www.ivanakajic.me