Lecturer: Philipp Wicke
Fields: Artificial Intelligence, Computational Linguistics, Language Models
Content
Most of the thousands of languages in the world share some key properties that enable us to exchange information within our language communities, but also beyond them. At the same time, we experience a shift in artificial intelligence, which heavily relies on the development of large language models for certain languages, which exhibit emergent, general human-like properties such as reasoning and planning. This course has a bipartite structure that combines the theory of robustness in languages from the perspective of cognitive science with the practice of responsible applications of generative language models.
* Session 1: Introduction to Language Robustness
In this introductory session, students will gain a profound understanding of language universals and the fundamental role they play in human communication. We explore universal properties of language and linguistic relativity, examining how language shapes our perceptions and thoughts.
* Session 2: Traits of Robust Language: Image Schemas and Embodiment
Building on the foundation laid in the previous session, we investigate the connection between language and conceptual understanding. Explore image schemas and embodiment as powerful mechanisms for robust concepts. Additionally, we introduce the concept of “Robustness of Concepts” and explore illustrative examples, including the fusion of language and emojis.
* Session 3: Large Language Models and Image Generation
This session opens up new horizons as we look at Large Language Models (LLMs). Learn about the possibilities and challenges of using these sophisticated models for various applications. We delve into multilingual studies involving Large Language Models, such as Glot500, and look at those concerned with embodiment and LLMs. We will also look at text-to-image generational models such as Midjourney, StableDiffusion or Dalle-2. Students will also gain insights into testing different models to identify their strengths and limitations.
* Session 4: Responsible Language Generation
Ethics takes center stage in this last session, as we focus on the responsible use of language generation technologies. We focus on the environmental impact of these models, considering factors such as emissions, energy consumption during training and inference, and e-waste generation and storage. Societal implications are also discussed, including bias in language models, data annotation through crowdsourcing, and the ethical challenges posed by deep fakes and fake news generation.
By the end of this course, students will have a comprehensive understanding of language universals, large language models, and ethical considerations.
Literature
- Evans, Vyvyan, and Melanie Green. Cognitive linguistics: An introduction. Routledge, 2018.
- Boroditsky, Lera. “Does language shape thought?: Mandarin and English speakers’ conceptions of time.” Cognitive psychology 43.1 (2001): 1-22.
- Wei, Jason, et al. “Emergent abilities of large language models.” arXiv preprint arXiv:2206.07682 (2022).
- ImaniGooghari, Ayyoob, et al. “Glot500: Scaling Multilingual Corpora and Language Models to 500 Languages.” arXiv preprint arXiv:2305.12182 (2023).
- Wicke, Philipp. “LMs stand their Ground: Investigating the Effect of Embodiment in Figurative Language Interpretation by Language Models.” arXiv preprint arXiv:2305.03445 (2023).
- Wicke, Philipp, and Marianna Bolognesi. “Emoji-based semantic representations for abstract and concrete concepts.” Cognitive processing 21.4 (2020): 615-635.
Lecturer
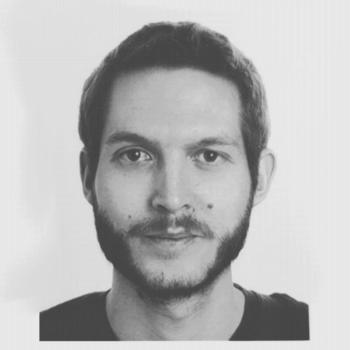
Philipp Wicke studied Cognitive Science at the University of Osnabrück in the B.Sc. programme. During these studies he interned at Dauwels Lab at the NTU Singapore in the field of neuroinformatics, he also interned at the Creative Language Systems Lab at UCD Dublin at which he later wrote his dissertation on “Computational Storytelling as an Embodied Robot Performance with Gesture and Spatial Metaphor” under supervisor Tony Veale. In his current role at LMU, Philipp is researching on Natural Language Processing and teaches programming in the B.A. and M.A. Computational Linguistics. Philipp Wicke is the Head of AI Applications of the AI for People Association and an Associate Member of the Munich Center for Machine Learning (MCML).
Affiliation: Center for Information and Language Processing (CIS), LMU – Munich
Homepage: www.phil-wicke.com